Please contact me if you are interested in selecting any of these projects.
DW-MSC-1
Implementation of a Multiscale Cellular-automata/ODE
model of the human colon.
Supervisors Dr Dawn Walker and Dr Bernard Corfe
Biological tissue is a multiscale system where subcellular level events influence cell behaviours, which in turn affect tissue structure and modify disease risk. The human colon is a particular example of this, where the tissue is composed of a large number of flask-like structures called crypts, which all contain individual biological cells, which divide, migrate and die. We have previously published an ODE (Ordinary Differential Equation) model of the colon crypt (Smallbone, K., & Corfe, B. M. (2014). A mathematical model of the colon crypt capturing compositional dynamic interactions between cell types. International Journal of Experimental Pathology, 95(1), 1-7). The model is available in xml: BIOMD0000000517.
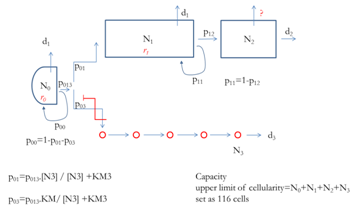
Cellular Automata is a computational paradigm that has been applied to the simulation of many real world systems. The system to be modelled is divided is represented as a lattice, with the properties of each lattice site (or geometric cell) at the next time step influenced by its current state and that of its neighbours.
The student will develop
a model of the colon, wherein each crypt is represented as a
single geometric
cell in a cellular automata model. The number of cells in this
crypt will be
informed by solving an ODE model . Rules
for interactions between crypts
within the CA will be developed in collaboration with the
supervisory
team. The overarching goal of the research team is to
develop a CA on the
scale of an entire colon or population. Over time and
with iterative
fitting to public health data the model will allow us to
understand better the
mechanisms of disease incidence.
The
project that will be undertaken by the student successfully
joining our team
will be to undertake critical components of the computational
development.
The aim of this project will be to:
i) write a cellular automata-based solver with Matlab or Python, representing multiple crypts in the colon.
ii) expand the functionality to allow an ODE to be solved in each geometric cell of the CA (crypt) at each time point.
ii) Carry out full testing of this model
from both a software
(unit testing) and modelling (sensitivity analysis)
perspective.
Pre-requisites - successful completion of COM6009 – Modelling and Simulation of Natural Systems is essential.
Programming in Matlab or Python
____________________________________________________________________________
DW-MSC-2 Developing a Java-based Genetic Algorithm to solve a Timetabling Problem
Genetic
algorithms (GAs) are a computational approach to solving
optimisation problems,
based on the process of DNA replication, crossover and
mutation drawn from
evolutionary biology.
An example
of a problem that can be tackled with such an approach is a
scheduling or
timetabling problem in a school or university. For instance
. in the Department
of Computer Science alone, there are many taught modules,
some of which may
include both undergraduate and postgraduate students, that
must be scheduled to
avoid clashes, in teaching rooms of various size, and
meeting various time
constraints of lecturers involved.
The objectives
of this project are as follows:
1.
To investigate the options for representing a scheduling
problem of this nature
in a GA format
2.
Implementation of a Genetic Algorithm approach to solve a
simplified version of
this problem (e.g. where room location of lecturer’s
constraints are ignored)
This can be
done either by writing code from scratch in Java or Python,
or adapting an
existing GA package to fit this particular problem.
Potential
extensions include
extending the approach to handle more complex problems (e.g.
taking into
account proximity of different teaching rooms or lecturer
availability).
Prerequisites: knowledge of
Java- or Python-based
programming. Previous experience of using Genetic Algorithms
would be an
advantage.
References
http://www.chem.eng.psu.ac.th/new_chem/upload/document/research/29/10.1.1.39.9345.pdf
Supervisors Dr Dawn Walker
and Dr Daniele Tartarini
Cancer is a complex disease that claims numerous lives every year. Recent post-genomic research has demonstrated that every cancer type responds differently to treatments and from patient to patient. It can spread to several organs during its evolution (a process known as metastasis). Therefore, the work of clinicians would be more effective if they could have a tool to make reliable diagnosis, prognosis that would pave the way for personalised treatments.
Cancer begins with some abnormal gene mutations in a cell that then starts to grow and divide without control. These mutations are present in all the cells of the cancer cell lineage thereby the analysis of their genetic profile could reveal useful information. In particular it has be shown in [1][2][6] that it is possible to use the genetic profile to classify cancer and understand the likelihood it will develop metastasis. The identification of this useful information in the genetic profile would allow the clinicians to better tailor the treatment.
A technique called gene expression profiling [3] allows researchers to identify the level of activity of genes within a cell. This activity profile, called a “gene expression profile”, provides a signature that can be used to classify tumors, which can impact on predicting the patient’s clinical outcome.
The aim of the project is to develop a tool to train a Multilayer Neural Network with back propagation (or Support Vector Machines) in order to recognise the sub-type of cancer (e.g. different types lung cancer) and its metastasis capability depending on the gene-expression profile. The tool will be validated using a cross-validation procedure and will be able to provide the prediction of the cancer type/status for new gene-expression profiles.
The gene expression data that will be used for training and validation is freely available on the Web as well as the libraries required to read them [4].
The tool will be developed using best practices: concurrent versioning system (git), unit testing, documentation and will be released open source.
Potential extensions to project: development of version of software in Cuda for Nvidia GPUs, or OpenMP for multicore architectures (including Intel Xeon Phi).
Requirements: Object oriented
programming language
recommended C++ (Python and Java can be considered),
familiarity with a
concurrent versioning system (git).
Attendance of module COM6509 Machine Learning and Adaptive
Intelligence is advised.
Optional skills: knowledge of machine learning algorithms.
Biological knowledge of cancer and gene expression is not a prerequisite.
References
[1] http://www.nature.com/doifinder/10.1038/ng1060
[2] http://www.tandfonline.com/doi/full/10.4161/sysb.25983#.VF_HLoeCVK0
[3] http://en.wikipedia.org/wiki/Gene_expression_profiling
[4] http://www.broadinstitute.org//cgi-bin/cancer/publications/pub_paper.cgi?mode=view&paper_id=80
[5] http://linkinghub.elsevier.com/retrieve/pii/S1044579X14000613
[6] http://www.nature.com/nm/journal/v7/n6/pdf/nm0601_673.pdf_________________________________________________________________
DW-MSC-4 Biofilm growth simulator on
GPU or multicore architectures
Supervisors Dr Dawn Walker and Dr Daniele Tartarini
Biofilms are everywhere both in natural and artificial settings. They may form on different types of surfaces: living tissues, medical devices, food storage and processing tools, water system piping, natural aquatic systems and generally speaking on any wet surface.
Biofilms generally consist of multi-species of bacteria, fungi, algae, yeasts, protozoa, other microorganisms, debris, corrosion products, and water. The development of a biofilm is a multistage process starting from water floating cells landing and attaching on a damp surface, multiplication to colonise the surface and excretion of adhesive proteins. They directly and indirectly cause costs of millions of pounds a year due to energy losses, equipment damage, food contamination and medical infections. Nevertheless they can be exploited as bio-barriers to protect soil from contamination, bio-filters for waste water and for remediate hazardous waste sites.
Mathematical or computational modeling of biofilms is therefore crucial to achieve a deeper understanding of the processes, verify experimental observations and predict the evolution of this complex microorganism.
The aim of this project is to develop a simulator of biofilm growth using an approach based on Cellular Automata. The simulator has to be able to accommodate different initial conditions: type of surface, nutrients distribution and different organisms types.
The student will optimise execution time using a multi-thread programming paradigm on multi core architectures: Nvidia CUDA with GPU, OpenMP with Intel Xeon Phi or a mainstream multicore CPU.
Potential extensions a graphical real-time representation of the simulation could be implemented using a state of the art graphic library.
Pre-requisites: Knowledge of Cellular Automata theory; Object oriented programming language with support for multithreading, ideally C++ but alternatively Python or Java can be considered (in which case the graphical representation would be required).
Skills desired or to be acquired during dissertation: multi-thread programming using OpenMP (on multicore CPU or Intel Xeon Phi) or Nvidia CUDA (on Nvidia GPU), unit test programming, performance analysis, distributed version control (git).
References to biofilm growth models
http://www.sciencedirect.com/science/article/pii/S0038109810000281
http://www.nature.com/nature/journal/v368/n6466/abs/368046a0.html
___________________________________________________________________________
DW-MSC-5 Tissue engineering and
wound healing simulator on GPU or multicore
architectures
Supervisors Dr Dawn Walker and Dr Daniele Tartarini
Tissue engineering is a branch of regenerative medicine that studies and develops bioartificial implants, tissue remodelling and repair, enhancement of tissue/organ functions.
This discipline has generated impressive achievements such as creating skin patches to heal wounds, and growing human tissue on bioscaffolds. Nevertheless a lot of theoretical and clinical research has to be done to improve the quality of artificial implants.
Tissue growth is a complex process whose pattern and rate depends on many factors like cell phenotype, nutrients concentration, scaffold shape, surface rugosity, cell density and spatial distribution. These factors can affect cell functions, proliferation, adhesion, migration, differentiation and they can be controlled through sophisticate biomaterial techniques.
Nevertheless to improve and validate these techniques expensive and time consuming experiments have to be performed.
The aim of this project is to create a software tool to simulate tissue growth in free 3D space, on simple scaffolds or in wound like geometries. The model used to simulate tissue growth will based on a 3D Cellular automata [1] and have to allow the user to choose different scaffold shapes and cell distribution as initial conditions.
The software will be developed using an object oriented language and following the best practices in software development: unit testing, documentation, concurrent versioning system.
The student will investigate the performance improvement implementing this simulator on Nvidia GPUs using CUDA, a multicore processor with OpenMP (or on the Intel Xeon Phi).
Ideally the project has to be developed in C++/CUDA or C++/OpenMP or alternatively languages like Python or Java can be used with a multi-threaded implementation. In all cases a parallel implementation has to be provided to compare performance improvement.
Prerequisites: object oriented programming, knowledge of cellular automata theory
Optional skills: knowledge of multithreading programming, CUDA or OpenMP. Attendance at COM6009 Modelling and Simulation of Natural Systems would be desirable.
References:
[1] http://www.ncbi.nlm.nih.gov/pmc/articles/PMC1367098/pdf/713.pdf
________________________________________________________________________
DW-MSC-6
Principal Component Analysis for cancer
classification on GPU/Multicore architectures
Supervisors Dr Dawn Walker and Dr Daniele Tartarini
Cancer begins with some abnormal gene mutations in a cell that then starts to proliferate without control. These mutations are present in all the cells of the cancer cell lineage thereby the analysis of their genetic profile would reveal useful information. In particular it has be shown in [1][2][5] that it is possible to use the genetic profile to classify cancer and understand the likelihood it will develop metastasis. The identification of this useful information in the genetic profile would allow the clinicians to better tailor the treatment.
A technique called gene expression profiling [3] allows to identify the level of activity of genes within a cell. This activity profile, called gene expression profile, provides a signature that can be used to classify cancer, which can impact on predicting the patient’s clinical outcome.
Understanding which genes carry the useful information to build this signature among the large number of genes available is a complex task. Different algorithms have been developed to identify the most significant genes to classify cancer by type/state and likelihood of developing metastases.
One the available approaches is based on Principal Component Analysis or PCA [5]. It allows researchers to identify the subset of the available genes that actually bring the minimum information to classify cancer. This would allow to reduce the data space dimensionality and potentially to visualise in a reduced dimension space. This approach is used to train machine learning classifiers in order to reduce the dimension of the feature vectors (gene expression) used during training. It reduces the complexity of the classifier and improves the classification accuracy.
The aim of this project is to develop a software tool to apply principal component analysis to a set of gene expression profiles. Considering the size of these datasets a multicore or GPU architecture will be used.
The software has to be developed using an object oriented language and following the best practices in software development: unit testing, documentation, concurrent versioning system.
The student will investigate the performance improvement implementing this simulator on Nvidia GPUs using CUDA, a multicore processor with OpenMP (or on the Intel Xeon Phi).
Ideally the project has to be developed in C++/CUDA or C++/OpenMP or alternatively languages like Python or Java can be used with a multi-threaded implementation. In all cases a parallel implementation has to be provided to compare performance improvement.
Optionally a predictor of cancer type can be implemented using an available library for Nvidia GPU.
Requirements: Object oriented programming language recommended C++ (Python and Java can be considered), concurrent versioning system (git).
Attendance of module COM6509 Machine learning and adaptive intelligence would be desirable.
Optional skills: machine learning algorithms. Biological knowledge of cancer and gene expression is not a prerequisite.
References:
[1] http://www.nature.com/doifinder/10.1038/ng1060
[2] http://www.tandfonline.com/doi/full/10.4161/sysb.25983#.VF_HLoeCVK0
[3] http://en.wikipedia.org/wiki/Gene_expression_profiling
[4] http://www.nature.com/nbt/journal/v26/n3/full/nbt0308-303.html
[5] http://www.nature.com/nm/journal/v7/n6/pdf/nm0601_673.pdf