Rob Gaizauskas : UG Level 3 Projects 2019-20
Email: Rob
Gaizauskas
Project Titles:
RJG-UG-1: | Aspect-based Sentiment Analysis
|
RJG-UG-2: | Size Matters: Acquiring Vague Spatial Size Information from Textual Sources
|
RJG-UG-3: | Character Identification in Multiparty Dialogues
|
RJG-UG-4: | Communicating with a Mobile Virtual Robot via Spoken Natural Language Dialogue
|
RJG-UG-5: | Natural Language Analysis in Scenario Based Training Systems
|
RJG-UG-6: | Using Deep Learning to Improve OCR for 19th Century British Newspapers
|
RJG-UG-7: | Building an Alexa Skill for DCS
|
[next][prev][Back to top]
RJG-UG-1:   Aspect-based Sentiment Analysis
Background
As commerce has moved to the Web there has been an explosion of on-line customer-provided content reviewing the products (e.g cameras, phones, televisions) and services (e.g. restaurants, hotels) that are on offer. There are far too many of these for anyone to read and hence there is tremendous potential for software that can automatically identify and summarise customers' opinions on various aspects of these products and services. The output of such automatic analysis would be of tremendous benefit both to consumers trying to decide which product or service to purchase and to product and service providers trying to improve their offerings or understand the strengths and weaknesses of their competitors.
By aspects of products and services we mean the typical features or characteristics of a product or service that matter to a customer or are likely to be commented on by them. For example, for restaurants we typically see diners commenting on the quality of the food, the friendliness or speed of service, the price, the ambience or atmosphere of the restaurant, and so on. The automatic identification of aspects of products or services in customer reviews and the determination of the customer sentiment with respect to them are tasks that natural language processing researchers have been studying for several years now. As is common in the field, shared task challenges -- community-wide efforts to define a task, develop data resources and metrics for training and testing and run a friendly competition to help identify the most promising approaches -- have appeared in recent years addressing the tasks of aspect identifcation and sentiment determinatio. Specifically, SemEval, an annual forum for the evaluation of language processing technologies across a range of tasks involving understanding some aspect of natural language, has run challenges on Aspect-Based Sentiment Analysis (ABSA) in 2014, 2015 and 2016.
The SemEval ABSA challenges provide a good place to start for any project on sentiment analysis as they supply: clear task definitions, data for training and testing, a conference proceedings with lots of discussion about different approaches and results of state-of-the-art systems to compare against.
Project Description
This project will begin by reviewing:
- existing frameworks for analysing sentiment and opinion in text
- existing algorithms for aspect-based sentiment analysis
- existing datasets for training and evaluation ABSA algorithms
- existing natural language processing and machine learning tools and toolkits, e.g. NTLK, Stanford Core and SciKit Learn.
Following this review, one or more approaches to ABSA will be chosen and implemented, building on existing NLP resources where it is sensible to do so. The resulting algorithm(s) will be evaluated using SemEval data and systes as benchmarks and refinements made to the algorithms, as far as time permits. Another line of possible work is to consider how best to summarise and present the results of an ABSA system to end users.
Prerequisites
An interest in natural language processing and machine learning and Python programming skills are the only prerequisites for the project.
Initial Reading and Useful Links
Contact supervisor for further references.
[next][prev][Back to top]
RJG-UG-2:   Size Matters: Acquiring Vague Spatial Size Information from Textual Sources
Background
How big is an adult elephant? or a toaster? We may not know exactly, but we have some ideas: an adult elephant is more than a meter tall and less than 10 meters; a toaster is more than 10cm tall and less than a meter. In particular we have lots of knowledge about the relative sizes of objects: a toothbrush is smaller than a toaster; a toaster is smaller than a refrigerator.
We use this sort of "commonsense" knowledge all the time in reasoning about the world: we can bring a toaster home in our car, but not elephant. An autonmous robot moving about in the world and interacting with objects would need lots of this kind of knowledge. Moreoever, we appear to use knowledge about typical object sizes, along with other knowledge, in interpreting visual scenes, especially in 2D images, where depth must be inferred by the observer. For example, if, when viewing an image, our knowledge about the relative sizes of cars and elephants is violated under the assumption that they are in the same depth plane, then we re-interpret the image so that the car or elephant moves forward or backward relative to the other, so that the assumption is no longer violated. Thus, information about relative or absolute object size is useful in computer image interpretation. It is also useful in image generation: if I want to generate a realistic scene containing cars and elephants then I must respect their relative size constraints. Various computer graphics applications could exploit such information.
Manually coding this kind of information is a tedious and potentially never ending task, as new types of objects are constantly appearing. Is there a way of automating the acquisition of such information? The hypothesis of this project is that there is: we mine this information from textual sources on the web that make claims about the absolute or relative sizes of objects.
Project Description
The project will explore ways of mining information about the absolute and relative size of objects from web sources, such as Wikipedia. The general task of acquiring structured information from free or unstructured text is called text mining or information extraction and is a well-studied application in the broader area of Natural Language Processing (NLP).
The project will begin by reviewing the general area of information extraction with NLP, with specific attention to tasks like named entity extraction (which has been used, e.g. to identify entities like persons, locations and organisation as well as times, dates and monetary amounts and could be adapted to identify object types and lengths) and relation extraction (which has been used to recognise relations between entities, such works-for, and attributes of entities, such as has-location, and could be adapted to recognise the size-of attribute).
Information extraction systems in general are either rule-based, where the system relies on manually-authored patterns to recognise entities and relations, or supervised learning-based where the system relies on learning patterns from manually annotated examples. Following initial reading and analysis of the data, a decision will be made about which approach to take.
In addition to identifying absolute size information (e.g. the Wikipedia article on "apple" says: "Commercial growers aim to produce an apple that is 7.0 to 8.3 cm (2.75 to 3.25 in) in diameter, due to market preference.") the project will also investigate how to acquire relative size information. For example, sentences indicating the topological relation inside ("You can help apples keep fresh longer by storing them in your pantry or refrigerator drawer") allow us to extract the information that apples are smaller than refrigerators.
By building up a set of facts expressing relative sizes, together with absolute size information, we can infer upper and lower bounds on the size of various objects. Of course, differring, even conflicting, information may be acquired from different sources. Some means of merging/reconciling this information will need to be devised.
The final element of the project will be to couple the acquired facts to a simple inference engine that will allow is to infer "best estimate" bounds on the size of objects from whatever facts we have acquired. E.g. if all we know is that apples fit inside refrigerators and that refrigerators are no more than 3m tall, then need to be able to infer that apples are less than 3m tall.
Of course, in addition to implementing the above capabilities the project will need to investigate ways of evaluating the various outputs. For example, the accuracy of identifying size information ("15 cm", "5-6 feet"), size-of information ( size-of(apple,7.0-8.3cm)) and relative size information ("apple <= refrigerator") needs to be assessed, as does the correctness of the inference procedure.
Prerequisites
Interest in language and/or text processing and in image analysis. No mandatory module prerequisities, but any or all of Machine Learning, Text Processing and Natural Language Processing are useful.
Initial Reading and Useful Links
- Jurafsky, Daniel and James H. Martin. (2008) Speech and Language Processing: An Introduction to Natural Language Processing, Computational Linguistics, and Speech Recognition (2nd ed). Prentice-Hall. Especially Chapter 22 on Information Extraction.
- Pustejovsky, J., J.L. Moszkowicz and M. Verhagen. 2011. ISO-Space: The annotation of spatial information in language. In Proceedings of the Joint ACL-ISO Workshop on Interoperable Semantic Annotation , 1--9.
- Jochen Renz (ed). (2002) Qualitative Spatial Reasoning with Topological Information. Springer. LNCS 2293.
- Pan, Feng, Rutu Mulkar-Mehta and Jerry R. Hobbs. 2007. Modeling and Learning Vague Event Durations for Temporal Reasoning. In Proceedings of the Twenty-Second Conference on Artificial Intelligence (AAAI-07).
[next][prev][Back to top]
RJG-UG-3:   Character Identification in Multiparty Dialogues
Background
Anaphora or anaphors are natural language expressions which "carry one back" to something introduced earlier in a discourse or conversation. In a conversation this could be a reference to one of the speakers or to someone else who is not a party to the conversation or to something else entirely.
For example, consider the following fragment from a dialogue in the well-known TV sitcom Friends:
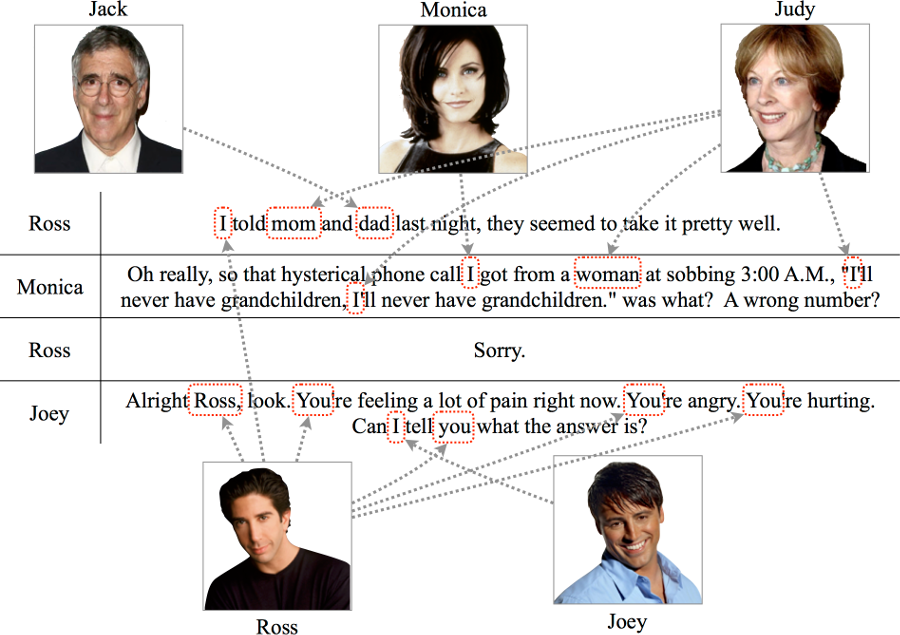
In the first sentence of this example, Ross uses the pronoun "I" to refer to himself and the common nouns "mom" and "dad" to refer to non-participants in the dialogue, Jack and Judy, who are the parents of Ross and Monica. Determining which linguistic expressions in a text or conversation refer to which real world entities, possibly introduced into the conversation by earlier linguistic expressions, is called the problem of reference resolution and is an important part of understanding the meaning of a conversation. Easy for humans, it is a significant challenge for computers.
The task of building reference resolution algorithms has been studied in Natural Language Processing (NLP) for some time. To develop systems and assess their performance the research community has built various annotated training and test datasets that contain manually annotated links between coreferring phrases or beteeen phrases and representations of the entities they refer to. In 2018 SemEval, a multi-year series of shared task evaluations of semantic analysis systems, ran an particular evaluation on Character Identification on Multiparty Dialogues. This evaluation challenged participants to build systems that could process dialogues from the Friends TV series and link mentions of entities in the dialogues to representations of the entity they are mentions of.
Project Description
This project's primary objective is to design, implement and evaluate a system to participate in the SemEval 2018 Task 4: Character Identification on Multiparty Dialogues. While the evaluation is officially over, it is still possible to register, download the resources, including the annotated training and test datasets as well as the scoring software.
The project will begin by reading background papers on the task and more broadly reviewing the academic literature in the area of reference resolution. The evaluation datasets and evaluation software will also need to be acquired and understood, particularly details of the annotation schemes. Several freely available coreference systems, specifically the Stanford and BART coreference systems, will be investigated to determine whether they could be use for this task.
The second part of the project will involve deciding on the approach to take and designing a system to participate in the challenge.
The third part of the project will involve the implementation and evaluation of the approach specified in the second part of the project, revising/improving the approach in the light of results as time permits.
Prerequisites
Interest in language and/or text processing and in image analysis. No mandatory module prerequisities, but any or all of Machine Learning, Text Processing and Natural Language Processing are useful.
Initial Reading and Useful Links
- Jurafsky, Daniel and James H. Martin. (2008) Speech and Language Processing: An Introduction to Natural Language Processing, Computational Linguistics, and Speech Recognition (2nd ed). Prentice-Hall. Especially Chapter 21 on Computational Discourse. E-copy in library.
- Choi, J.D. and H. Y. Chen, SemEval 2018 Task 4: Character Identification on Multiparty Dialogues (2018). Proceedings of the International Workshop on Semantic Evaluation, SemEval'18, 57-64, New Orleans, LA.
- Chen, H.Y., E. Zhou, and J. D. Choi (2017). Robust Coreference Resolution and Entity Linking on Dialogues: Character Identification on TV Show Transcripts. Proceedings of the 21st Conference on Computational Natural Language Learning, CoNLL'17, 216-225 Vancouver, Canada.
- Chen, H.Y. and J. D. Choi (2016). Character Identification on Multiparty Conversation: Identifying Mentions of Characters in TV Shows. Proceedings of the 17th Annual SIGdial Meeting on Discourse and Dialogue, SIGDIAL'16, 90-100, Los Angeles, CA.
- The Stanford Coreference System
- The Beautiful Anaphora Resolution Toolkit (BART)
[next][prev][Back to top]
RJG-UG-4:   Communicating with a Mobile Virtual Robot via Spoken Natural Language Dialogue
Background
Robotics is a field of huge intellectual challenge with immense practical potential, increasingly attracting the attention of industry, government as well as academia. As robots begin to appear more and more in daily life, a central issue will be communication between humans and robots.
One approach to communicating with robots that has huge potential is spoken natural language dialogue, especially in humn-robot co-working environments.
Researching spoken dialogue systems (SDS) for human-robot co-working is a complex endeavour. One important prerequisite is that we have available a lab-based setup where humans can interact with virtual robots, which are mucher easier and cheaper to work with than real robots, so that dialogue researchers can develop and test computational models of dialogue. One widely-used platform for developing robot software is ROS -- Robot Operation System. It, together with Gazebo (a simulation package for robots that allows visualization of a robot interacting with a virtual environment), form an ideal environment for developing and demonstrating robot behaviours that can later be straightforwardly moved onto real physical robots. In particular it could form a very powerful setting for human-robot spoken dialogue research. However, at present there are no spoken language recognition or generation tools integrated into the ROS platform nor has there been much experimentation with spoken language dialogue in virtual robot environments.
A previous Y3 project has developed software within the ROS framework that allows a virtual robot to localise itself and navigate in a virtual world. Thus tools to build a virtual world, create a map of it and allow a robot to navigate within it are available. Efforts to secure funding to integrate speech recognition and generation capabilities, using existing open source libraries, within ROS are underway and progress to achieve this may have been made by the time this project starts.
Project Description
The aims of this project are two-fold:
- To complete the integration of speech recogniton and generation components within ROS, depending on their state of integration at the time the project commences, and to create a ROS package for speech recognition and genertion that others could use in the future;
- To develop a dialogue manager that will allow a user to use spoken language to direct a mobile robot around
a simple virtual world where the robot may interact with the user to answer questions about whether it can sense something or to ask questions to clarify ambiguous commands. This will provide an experimental setting to explore the research challenges in getting humans and robots to establish, via spoken dialogue, common points of reference in the shared virtual world, getting the robot to interpret spatial instructions, etc.
At the end of the project the student should have a solid understanding of the basics of developing software on the ROS platform, of using speech recognition and generation libraries and of the challenges involved in developing dialogue systems.
Prerequisites
Interest in robotics and in spoken natural language dialogue. The client libraries will be using in ROS are Python-based and geared towards Unix/Linux, so student should be comfortable with this.
Initial Reading and Useful Links
- Chapter 25 in Russell, Stuart and Norvig, Peter
(2010) Artificial Intelligence: A Modern Introduction (3rd
ed). Pearson.
(e-book available from University Library)
- Jurafsky, Daniel and James H. Martin (2008). Speech and Language Processing: An Introduction to Natural Language Processing, Computational Linguistics, and Speech Recognition (2nd ed). Prentice-Hall. Especially Chapter 24 on Dialogue and Conversational Agents.
- Wikipedia article on Robot Operation System
[next][prev][Back to top]
RJG-UG-5:   Natural Language Analysis in Scenario Based Training Systems
Note: This project offers the chance to work with Certus Technology Associates, a company that "designs, builds, delivers and supports adaptable software and services, to assist higher education establishments, life-science, biotech, healthcare and private sector organisations in the management of complex data and processes." Certus is interested in exploring the potential natural language processing (NLP) has to improve their software products and services. Any student taking this project will have the chance to meet with Certus personnel to refine requirements, acquire sample data and to get feedback on their work.
Background
Certus has developed a training and competence system where a user
interacts with a virtual environment to complete a task. On request, the
system generates a complex, randomised training scenario based upon a
constrained domain model and a set of probability functions. The user
interacts with this new training scenario and, on completion, their
interaction is immediately evaluated and their performance scored.
For example, in one application the system is used to create a scenario
of a biomedical scientist working in a blood transfusion laboratory. In
the virtual laboratory, a sample reception in-tray contains a patient
blood sample and a referral card requesting the blood be tested and 2
units of matched plasma be made available for surgery planned for later
in the week.
The user of the training system first reviews the referral and then
moves between scenes in the virtual lab in the normal way to complete
the request. This includes putting the blood through an analyser and
interpreting results, evaluating blood type and antibody content and
selecting appropriate blood products from a stock fridge.
In the blood transfusion training system, there are a number of scenes
to which the user can navigate. These mimic the real working
environment. They include a telephone, an email programme and a
laboratory log book. For such scenes, free text is entered by the user.
For example, if the user finds that the patient name printed on the
referral card does not match that written on the blood sample tube, they
should send a message to the ward requesting a new patient sample. They
should provide a reason.
Project Description
The objective of this project is to investigate potential NLP approaches
to extracting enough semantics from free text entries entered in a
scenario participation to enable evaluation of the appropriateness of an
action. Such evaluation is to be based upon the text entered by the
user, information about the scenario, and a set of evaluation rules.
Scenarios involving free text analysis include:
- Calling a ward
- Emailing a clinician
- Making notes on a variant
- Explaining a blood product selection
The project will involve:
- meeting with the client (Certus) to further determine their requirements and acquire data for training and testing;
- reviewing existing NLP techniques that may be appropriate as part of an automatic evaluation process within the proposed training system, e.g. information extraction techniques such as entity recognition and relation extraction;
- choosing the most appropriate techniques for the problem at hand and then designing and implementing a prototype system to
Prerequisites
Interest in language and/or text processing. No mandatory module prerequisities, but any or all of Machine Learning, Text Processing and Natural Language Processing are useful. Python is likely to be the language used to implement the system.
Initial Reading and Useful Links
- See supervisor for suggested readings.
[next][prev][Back to top]
RJG-UG-6:   Using Deep Learning to Improve OCR for 19th Century British Newspapers
Background
This project will take place within the context of a collaboration between the Departments of Computer Science and History at the University of Sheffield. The Department of History has in previous projects created a database, called the Digital Panopticon, of "life archives" of convicts in late 18-th to early 20th century in Britain and Australia. They now wish to supplement the information in the Digital Panopticon by automatically extracting information about crimes and police court trials from English newspapers of the period and linking it to the relevant records in the Digital Panopticon.
This information extraction/record linkage project poses numerous technical challenges, many of which are highly relevant to government and corporate orgonisations today. These challenges include:
- working with "noisy" text derived via optical character recognition (OCR) from images of historic newspapers
- working with historic English with many differences from modern day English and with many mis-spellings or olternate spellings
- addressing the "normal" challenges of information extraction from text including recognising and classifying entities (persons, places, dates, etc.) and relations (where someone lives, their profession, when they were convicted)
- linking someone mentioned in a newspaper coverage of a police court trial with the correct entry in the existing archive, which contains records for many individuals with the same name or similarly spelt name.
These challenges are far too many and difficult for one Y3 project. Consequently this project will address just the first: improving the quality of the existing OCR'ed representation of the source newspapers.
Project Description
The aim of this project is to explore whether and how the quality of optical character recognition over 19th century British newspapers can be improved using deep learning techniques. As inputs we have the (1) images of a large volume of 19th century British newspapers (2) the results of some unspecified errorful OCR process which has been run over the newspapers by a third party (call this "the newspaper dataset"). The goal is to see whether the quality of the output OCR text can be substantially improved, as at present it is a significant issue for the overall project (described above) which relies on accuracy of the OCR process. One open question is whether to ignore the existing OCR output and start from scratch or to run a corrective postprocess over the existing OCR output or to use a combination of these two techniques.
One of the earliest applications of recursive neural networks (RNNs) was to OCR and, with recent advances in deep learning, they seem to be a highly promising avenue to explore in addressing the central challenge of the project.
The project will procced by:
- reviewing existing approaches to OCR and to RNN approaches in particular
- reviewing existing toolkits for deep learning, such as TensorFlow and PyTorch
- developing training and testing data for the newspaper dataset
- design, implement and test one or more approaches to generating improved OCR output for the newspaper dataset
- evaluate the system(s) developed in the preceeding step
Prerequisites
Interest in neural networks and language processing. No mandatory module prerequisities, but any or all of Machine Learning and Text Processing and are useful.
Initial Reading and Useful Links
[next][prev][Back to top]
RJG-UG-7:   Building an Alexa Skill for DCS
Background
In a move to open the Amazon Alexa platform to third party developers -- as Apple and Google have opened their mobile device platforms to App developers -- Amazon has created the concept of a "Skill" (like an App) and a development kit to support independent developers in building skills. Amazon says:
"Alexa provides a set of built-in capabilities, referred to as skills. For example, Alexa's abilities include playing music from multiple providers, answering questions, providing weather forecasts, and querying Wikipedia.
The Alexa Skills Kit lets you teach Alexa new skills. Customers can access these new abilities by asking Alexa questions or making requests. You can build skills that provide users with many different types of abilities. For example, a skill might do any one of the following:
- Look up answers to specific questions ("Alexa, ask tide pooler for the high tide today in Seattle.")
- Challenge the user with puzzles or games ("Alexa, play Jeopardy.")
- Control lights and other devices in the home ("Alexa, turn on the living room lights.")
- Provide audio or text content for a customer's flash briefing ("Alexa, give me my flash briefing")"
(from Build Skills with the Alexa Skills Kit)
Just how popular and widespread skills will become is not clear yet. But clearly spoken language conversational interfaces are only going to become more widely used in the coming years. For any organisation wanting to get information about itself across to its "customers" a dedicated skill provides a new channel by which to do so. As a Computer Science Department with leading Speech and Language Processing research groups, it seems obvious that DCS at Sheffield should promote ourselves in this way.
Project Description
This aim of this project is to build upon three projects run in 2018-19 that created an Alexa Skill for the Department of Computer Science here at Sheffield. Those projects' aim was to build an Alexa Skill that would let interested persons converse with Amazon's Alexa to ask questions and get answers about the Department. For example, prospective students may want to know about courses offered; current students may want to know about lecture locations, and so on.
The current project will involve:
- getting an understanding of how dialogue systems work by reviewing the relevant literature
- understanding the Alexa Skills developer kit
- analysing the existing projects' outputs with a view to understanding what they have accomplished and how the skill can be move forwards towards becoming something the Department could actually deploy, specifically how to
- extend its coverage
- make its dialogues more natural
- improve its maintainability
- carry out further user studies
- designing, implementing and testing the enhanceements identified in the analysis stage
- evaluating the enhanced system with some candidate users
Prerequisites
Interest in speech and language processing. No mandatory module prerequisities, but any or all of Bio-Inspired Computing, Text Processing and Natural Language Processing are useful.
Initial Reading and Useful Links
Last modified May 12 2019 by Rob Gaizauskas